Recently, the international academic journal Remote Sensing of Environment published a joint research paper by the Wuhan University’s Satellite Navigation and Positioning Technology Research Center, the Institute of Artificial Intelligence and the University of New South Wales in Australia online ----information fusion for GNSS-R wind speed retrieval using statistically modified convolutional neural network.
Guo Wenfei, the associate professor of the Satellite Navigation and Positioning Technology Research Center, is the first author of the paper. Professor Guo Chi is the corresponding author. The Institute of Artificial Intelligence is the communication unit, and Du Hao, a postgraduate, participated in the research.
GNSS-R is a new crossing technology that integrates satellite positioning and quantitative remote sensing. One of its typical applications is to use radio signals such as GNSS navigation satellites to reverse the changing law of wind speed of global sea surface. At present, most GNSS-R wind speed reverse algorithms extract signal features from delay-Doppler images (DDM) artificially. Based on human experience, the association between these characteristics and ocean wind speed is established. But the results are greatly affected by various factors and the reverse accuracy is low, which greatly reduces the practical value of GNSS-R.
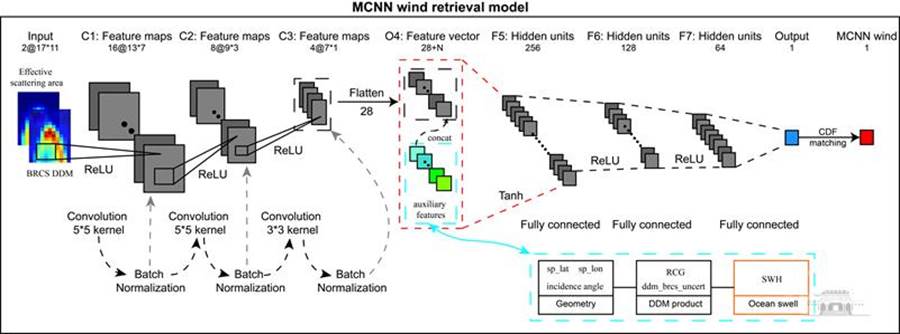
Figure 1 convolution neural network model based on cumulative distribution function correction
The research put forward a technical approach of integrating artificial intelligence, satellite navigation and remote sensing, and a convolution neural network model based on the correction of cumulative distribution functions was designed (Figure 1). The model employs end-to-end artificial intelligence network adaption to extract the effective features in DDM, and integrates the advanced auxiliary information of effective waves to obtain preliminary wind speed reverse results. The research makes full use of the advantages of deep learning technology, which can effectively integrate any parameters that impact sea surface wind speed reverse, so as to found a complete and robust wind speed reverse model and bring breakthrough technology changes to this field.

Figure 2 space-time performance of reverse wind speed
The experimental results show that taking the ECMWF ERA5 10-meter wind speed product as an example and with 17-month American CYGNSS V2.1 data, the average square root error of the model reverse wind speed in the range of 0-25 m/s is 1.53m/s, and the system deviation is -0.097m/ S, significantly excelling other existing methods. The study also analyzes the space-time performance of the proposed model reverse wind speed (Figure 2). The results indicate that the reverse wind speed is in good spatial consistency with ERA5 wind speed products, and the reverse wind speed does not drift with time.
This work is in collaboration with the Andrew G. Dempster’s research group, the professor at the University of New South Wales, and is funded by the National Natural Science Foundation (41604021, 41974031).
It is known that the Satellite Navigation and Positioning Technology Research Center is one of the important co-construction sites of the School's Artificial Intelligence Research Institute. Over the years, it has been committed to cutting-edge innovative research of interdisciplines, especially in the fields such as GNSS-R, robot unmanned systems, high-precision positioning timing and so on to enormously promote the integration of artificial intelligence technology.
Passage link: https://doi.org/10.1016/j.rse.2022.112934
Rewritten by Xie Anqing
Edited by Guo Chi,Wang QunEdited by Cao Mi and Hu Sijia